BY MATT BUCKLEY
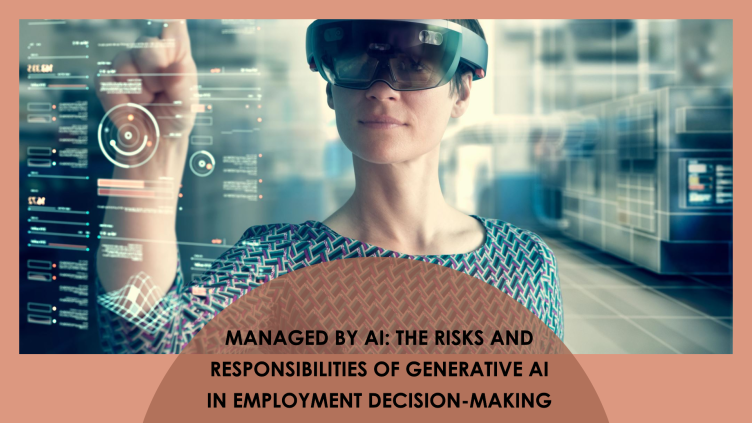
Management consulting firm McKinsey noted in 2023 that “a chatbot may not take your job—but it will almost certainly change it”. The notion that generative AI applications such as these will radically transform the world of work has become an increasingly dominant perspective in the field, and one largely upheld by a recent International Labour Organisation (ILO) report on “Generative AI and Jobs”. This transformation will not just come in the form of more productivity and improvements in efficiency but will impact the daily lives of workers everywhere; the question we must ask ourselves is how workers’ rights, the safety of the workplace, and quality of work for those using AI can not only be best safeguarded, but also advanced?
Generative AI is expected to be widely adopted in recruitment, administrative, and human resources roles, yet training on the risks posed by misuse or even “correct” use of these tools, both on employees and potential recruits, can lag behind adoption. Even advanced modern generative AI systems have many technical and contextual limitations, and pushing such systems beyond these operational limits can cause serious detriment to employees, potential recruits, and enterprises themselves. Examples of “intelligent systems” causing significant harm via use outside their design constraints have been recorded as far back as 2010, with the (temporary) loss of $1 trillion in market value during the “flash crash” of 6th May.
Understanding and Context
Current generative AI systems do not possess or create an encoding of the meaning of what they produce, only how words relate to one another linguistically, meaning such systems can and do blindly copy assumptions expressed in their training data; only humans have the ability to understand language, at least for the foreseeable future. The vast quantities of textual data required to train models like GPT-4 mean that attempts to filter datasets, particularly high-quality datasets, are often balanced against performance to maximise data availability, and it’s not only offensive words or phrases that can slip through. There are multiple documented examples of ChatGPT making stereotyped assumptions about the gender of medical professionals; how many proofreaders would be able to identify these gendered assumptions in a hastily generated job description without knowledge of the risks and what to look out for? Access to training in the responsible use of LLMs in recruitment processes is a vital part of any responsible AI usage policy.
Automation Bias
Automation bias is the shared propensity to overly rely on the recommendations of automated decision-making systems, even where contradictory information is available. It should be a core concern of any use of AI in employment decision-making, particularly where human review is required (i.e. at almost every stage). A policy approach that relies on manual review to validate high-risk recommendations, such as those directly impacting employment and compensation, must take into account how users interact with the system in practice. A recent and pertinent example would be the UK Post Office’s Horizon scandal, with hundreds of innocent employees being convicted of crimes by their employer using evidence from a computer system that was known to be unreliable – but this fact could not overcome the misplaced trust employees, and especially senior executives, placed in the system. A similar type of assumption about technology can take hold at an organisation level, particularly one in which the capabilities of systems are overestimated, and their weaknesses are not well-understood. New approaches that consider these aspects of human psychology, such as evaluative AI, are an active area of research, but a responsibility-first AI culture need not inhibit innovation.
Building a Responsible AI Policy
It is important for SMEs to actively consider how they should use AI in employment decision-making. The RAISE project has developed an initial set of guidelines to help SMEs deploy generative AI responsibly, but these cannot cover every use case. Active communication and consultation with employees and unions, where applicable, is an important (and in some jurisdictions, legally required) part of technology implementation plans, particularly those that use employee data or are involved in employment decision-making. In the UK, the recently published Artificial Intelligence (Regulation and Employment Rights) Bill presents one potential legislative framework. As proposed by the Trade Union Congress (TUC), the Bill builds upon existing employment legislation whilst taking the approach of the UK General Data Protection Regulation (GDPR) in applying universal human rights, such as the right to privacy, to the AI and data space. For example, Part 3 outlines requirements for Workplace AI Risk Assessments, including “an assessment of the risks to the rights of workers, employees or jobseekers” under a number of other pieces of legislation, including the Human Rights Act and the GDPR itself. Others are in development, and it’s those enterprises that implement responsible AI principles today that will attract the best employees, that will benefit from reduced compliance costs as national and international regulation is introduced, and most importantly of all, that demonstrate their commitment to valuing both human involvement and innovation.